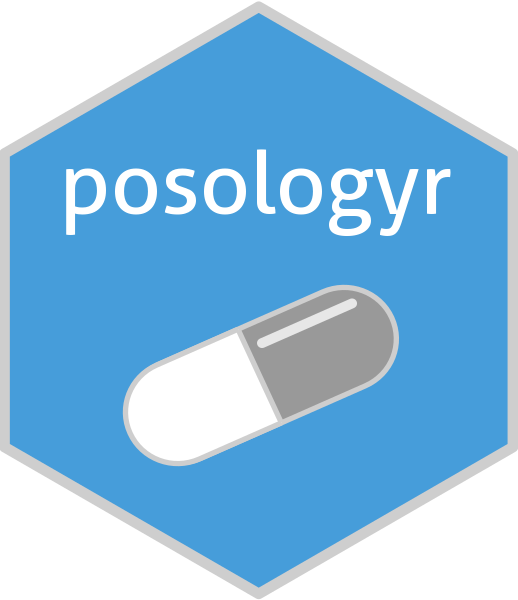
Estimate the posterior distribution of individual parameters by MCMC
Source:R/param_estim.R
poso_estim_mcmc.Rd
Estimates the posterior distribution of individual parameters by Markov Chain Monte Carlo (using a Metropolis-Hastings algorithm)
Arguments
- dat
Dataframe. An individual subject dataset following the structure of NONMEM/rxode2 event records.
- prior_model
A
posologyr
prior population pharmacokinetics model, a list of six objects.- return_model
A boolean. Returns a rxode2 model using the estimated ETAs if set to
TRUE
.- burn_in
Number of burn-in iterations for the Metropolis-Hastings algorithm.
- n_iter
Total number of iterations (following the burn-in iterations) for each Markov chain of the Metropolis-Hastings algorithm.
- n_chains
Number of Markov chains
- nocb
A boolean. for time-varying covariates: the next observation carried backward (nocb) interpolation style, similar to NONMEM. If
FALSE
, the last observation carried forward (locf) style will be used. Defaults toFALSE
.- control
A list of parameters controlling the Metropolis-Hastings algorithm.
Value
If return_model
is set to FALSE
, a list of one element: a
dataframe $eta
of ETAs from the posterior distribution, estimated by
Markov Chain Monte Carlo.
If return_model
is set to TRUE
, a list of the dataframe of the posterior
distribution of ETA, and a rxode2 model using the estimated distributions of
ETAs.
References
Comets E, Lavenu A, Lavielle M. Parameter estimation in nonlinear mixed effect models using saemix, an R implementation of the SAEM algorithm. Journal of Statistical Software 80, 3 (2017), 1-41.
Examples
# model
mod_run001 <- function() {
ini({
THETA_Cl <- 4.0
THETA_Vc <- 70.0
THETA_Ka <- 1.0
ETA_Cl ~ 0.2
ETA_Vc ~ 0.2
ETA_Ka ~ 0.2
prop.sd <- sqrt(0.05)
})
model({
TVCl <- THETA_Cl
TVVc <- THETA_Vc
TVKa <- THETA_Ka
Cl <- TVCl*exp(ETA_Cl)
Vc <- TVVc*exp(ETA_Vc)
Ka <- TVKa*exp(ETA_Ka)
K20 <- Cl/Vc
Cc <- centr/Vc
d/dt(depot) = -Ka*depot
d/dt(centr) = Ka*depot - K20*centr
Cc ~ prop(prop.sd)
})
}
# df_patient01: event table for Patient01, following a 30 minutes intravenous
# infusion
df_patient01 <- data.frame(ID=1,
TIME=c(0.0,1.0,14.0),
DV=c(NA,25.0,5.5),
AMT=c(2000,0,0),
EVID=c(1,0,0),
DUR=c(0.5,NA,NA))
# estimate the posterior distribution of population parameters
poso_estim_mcmc(dat=df_patient01,prior_model=mod_run001,
n_iter=50,n_chains=2)
#>
#>
#> Error : cannot convert to rxUi object
#>
#>
#> Error : cannot convert to rxUi object
#> Error in priormod$ppk_model: object of type 'closure' is not subsettable